Melbourne reopens today with a significant lift in restrictions and promises of more to pave our way back to the freedoms we had before this pandemic!
Are you energised by the prospect of accelerating projects that have lost some momentum?
Or perhaps frustrated and wanting a return into offices to revive projects that are 6-18 months behind on targets?
If your projects have a dependency on Data, here are a couple of key decisions that you can make to help your projects accelerate over the next 12 months!
People and Technology
It’s no secret that the IT sector has a squeeze on resources right now. Don’t just fill your current vacancies. This is your chance to re-evaluate if you truly need another Data Engineer. Here’s how you can tell:
- Your products are not shipping any faster. In fact, they’ve never truly accelerated.
- The design phase takes a long time, it always has
- Your teams iterate substantially (too much) to reach an MVP
If the above resonates, it’s time to consider repurposing some of your vacancies. Perhaps your bottleneck isn’t in development.
By the way, the answer is usually not more software or technology.
Choose features that help your teams accelerate
We have a habit of looking at the end-goal as the prize and the prize tells us we need campaign analysts, decisioning specialists, data scientists, full stack devs, data engineers. Well yes… but these roles can’t accelerate if their ecosystem is complex and making sense of the ecosystem takes months at a time.
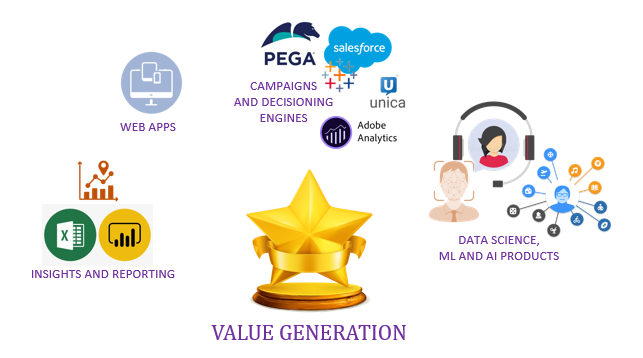
Consider investing in one or all of these data products instead. These will accelerate your delivery and help your teams scale.
- Integrated Enterprise Model: Not just a knowledge asset but an actual data repository that integrates your organisation’s data and crucially, describes it relative to the processes that it’s derived from. It is high quality detailed data – for a myriad of use cases.
- Subject Centric Models: Not just a knowledge asset but an actual data mart that prepares the data consistently for your highest priority areas.
- Feature Models: And finally, for your data science, machine learning and AI products – models already pivoted to the common features that your data scientists need. These help reduce the weeks (sometimes months!) of data wrangling that your data science teams perform.
For the above, you will need sense-makers of your data. They come as Data Architects, Data Modellers and Data Analysts in the Information Management domain. Word to the wise: these are not architects, modellers and engineers in application domains.
And note – these assets can be built progressively as well. You will need to give your teams a headstart to lay down some groundwork, but clever prioritisation aligned to business priorities will mean that your teams will realise the economies of scale that come from ready data assets.
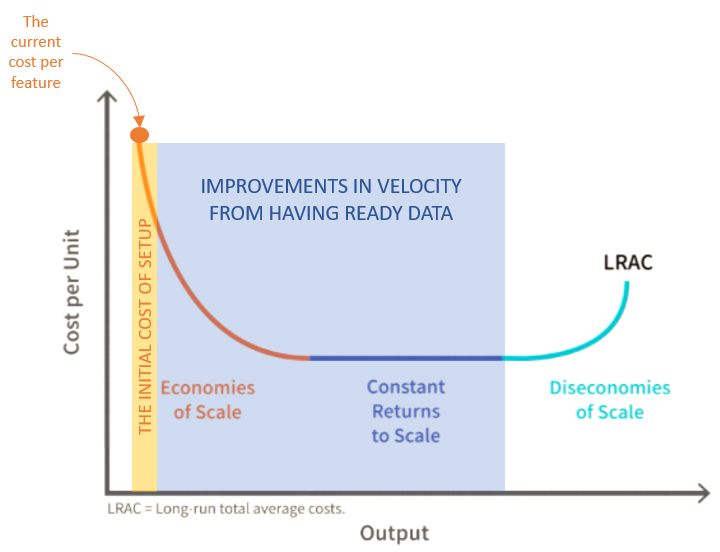
I hope these tips help you smash some goals and turn around your projects this next year! And hey, we’re a friendly bunch. Please reach out if you’d like to talk through the nitty gritty of accelerating your data projects.